views
Smart Availability: Unlock Business Growth with Data
In today’s fast-paced and competitive corporate world, how an organization distributes its resources and prepares its offerings can have a major impact on its financial performance. Anticipating the readiness of products or services is no longer just a tactical move—it’s a strategic imperative. For companies aiming to enhance operational flow, refine capacity utilization, and raise the bar on customer experience, availability forecasting has emerged as a vital tool in their decision-making arsenal. By leveraging past trends, deploying cutting-edge predictive models, and incorporating machine learning, businesses can forecast availability with impressive precision—driving consistent and scalable growth.
This piece explores the deeper dimensions of availability forecasting, shedding light on why it’s essential, how it works, the hurdles organizations commonly encounter, and where it’s headed next. It also includes practical takeaways for businesses eager to sharpen their competitive edge in a marketplace that’s constantly evolving.
Understanding Availability Forecasting
Availability forecasting is a structured approach used to predict when goods or services will be accessible, using a mix of data analytics and statistical modeling. It’s an essential practice in sectors like manufacturing, logistics, and retail, where efficiency hinges on timing and demand alignment. With accurate forecasts, companies can better manage stock, reduce the risks of shortages or excess inventory, and synchronize supply operations with actual demand—all while keeping costs in check.
Fundamentally, availability forecasting can be viewed as a specialized extension of demand forecasting, with a focus on aligning anticipated customer requirements with production or delivery capabilities. This alignment is achieved by diving deep into historical records, examining market signals, adjusting for seasonal demand shifts, and keeping a pulse on consumer trends—helping businesses scale smartly and stay nimble amid ever-changing conditions.
Provide Real-Time Availability Forecasting with ToolkitX’s Availability Forecasting software - https://toolkitx.com/campaign/availability-forecasting/
The Importance of Availability Forecasting in Modern Business
The benefits of availability forecasting extend far beyond merely keeping shelves stocked or production lines running. Here are some key reasons why this process is essential:
- Enhanced Operational Efficiency:
By integrating availability forecasting into everyday operations, companies can fine-tune their supply chains to reduce waste and cut operational costs. Maintaining balanced inventory levels means fewer idle resources and minimized storage expenses. - Improved Customer Satisfaction:
When companies forecast product availability accurately, they can avoid stockouts and delays—ensuring that customers always get what they need when they need it. This results in higher customer loyalty and repeat business. - Strategic Resource Allocation:
Forecasting provides insights that enable companies to allocate resources where they are most needed. Whether it’s investing in additional production capacity or scheduling labor shifts more efficiently, well-informed decisions lead to better overall performance. - Risk Mitigation:
Effective forecasting helps identify potential disruptions in the supply chain early on. Businesses can then implement contingency strategies to mitigate risks, ensuring business continuity.
Key Techniques and Methods for Availability Forecasting
Availability forecasting is rooted in a blend of traditional methodologies and modern technological advancements. Here are some of the common techniques used by industry experts:
1. Statistical Analysis and Time-Series Forecasting
This method involves the examination of historical data to detect patterns and trends over time. Time-series analysis can be particularly effective in industries with pronounced seasonal cycles or predictable fluctuations.
- Techniques include:
- Moving averages
- Exponential smoothing
- ARIMA (AutoRegressive Integrated Moving Average)
2. Predictive Analytics and Machine Learning
With the advent of big data, predictive analytics has taken center stage in availability forecasting. Machine learning algorithms sift through vast amounts of data to pinpoint correlations that traditional methods might miss. These systems are self-improving and adapt to changes in real time.
- Techniques include:
- Regression models
- Decision trees
- Neural networks
- Ensemble methods
3. Simulation and What-If Scenarios
Many organizations use simulation models to test different scenarios. By creating virtual models of various supply chain configurations or market conditions, companies can identify the most efficient strategies to forecast availability.
- Techniques include:
- Monte Carlo simulations
- Scenario analysis
- Sensitivity analysis
Challenges in Availability Forecasting
Despite its advantages, availability forecasting comes with its own set of challenges that can complicate the process:
Data Quality and Integration
Reliable data is the foundation of accurate forecasting. Many organizations struggle with fragmented systems where data is stored in silos. Integrating these disparate data sources into a unified system can be a significant hurdle.
Dynamic Market Conditions
Market conditions can change rapidly due to unforeseen factors such as economic shifts, natural disasters, or geopolitical events. This volatility can render even the most sophisticated models less reliable.
Technological Complexity
Implementing machine learning algorithms and predictive models requires a deep understanding of both data science and the specific industry context. Finding and retaining the right talent can be challenging and costly.
Balancing Over- and Under-forecasting
Striking the right balance between having too much inventory (leading to waste) and too little (resulting in missed sales opportunities) remains a perennial challenge for businesses.
Best Practices for Optimizing Forecast Accuracy
To overcome the challenges mentioned above and enhance the reliability of availability forecasts, consider implementing these best practices:
Utilize Integrated Data Platforms
Adopting platforms that centralize data from multiple sources can help improve forecast accuracy by ensuring a more comprehensive view of operations.
Invest in Advanced Analytics Tools
Modern analytics tools incorporating AI and machine learning capabilities can continuously update and refine forecasting models based on real-time data.
Regular Model Calibration
Forecasting models must be recalibrated regularly to adapt to new market trends and data anomalies. This ensures that forecasts remain relevant and accurate over time.
Foster Cross-Department Collaboration
Encourage communication and collaboration between sales, marketing, supply chain, and finance teams. This cross-functional approach can provide richer context and improve forecasting insights.
Implement Contingency Planning
Always have a backup plan. By building flexibility into your forecasting process, you can quickly adapt to unexpected changes and mitigate potential risks.
Future Trends in Availability Forecasting
As technology evolves, so too will the methods and applications of availability forecasting. Here are some emerging trends to watch:
Real-Time Data Integration
With the increasing Internet of Things (IoT) penetration, real-time data integration is becoming feasible. This means availability forecasts can be adjusted on the fly based on instantaneous changes in production, sales, or logistics.
Greater Use of Big Data
Big data technologies will continue to enhance forecasting accuracy by providing a more granular, real-time view of market conditions and consumer behavior.
Enhanced Predictive Modeling
As machine learning algorithms become more advanced, predictive models will improve, offering more precise insights into future availability scenarios. This will further enable businesses to optimize inventory and capacity planning.
Increased Emphasis on Sustainability
Businesses are now considering sustainability as a core factor in forecasting. Availability forecasting models increasingly account for environmental impacts and ethical sourcing, making the predictions more holistic.
Availability forecasting is transforming how companies manage their operations, reduce costs, and enhance customer experiences. By leveraging a mix of statistical methods, predictive analytics, and advanced technology, businesses can navigate complex market dynamics and achieve superior operational efficiency. As we move into an era characterized by real-time data and fast-evolving market conditions, staying ahead means continually refining forecasting models and embracing new technologies.
For businesses aiming to secure a competitive edge, investing in robust availability forecasting systems is not just an operational necessity—it’s a strategic imperative for future growth.
FAQs on Availability Forecasting
- What is availability forecasting and why is it important for businesses?
Availability forecasting is the process of predicting the future availability of products or services using historical data, statistical methods, and machine learning techniques. It is important because it helps businesses optimize inventory, reduce costs, and ensure better customer satisfaction. - How does predictive analytics enhance availability forecasting?
Predictive analytics leverages advanced algorithms and machine learning to analyze historical and real-time data. This enhances forecasting accuracy by identifying trends, seasonal patterns, and potential anomalies that traditional methods might miss. - What are the main challenges in implementing availability forecasting?
Key challenges include data quality and integration, rapidly changing market conditions, technological complexity, and balancing the risk of over- or under-forecasting. Addressing these challenges requires integrated data systems and advanced analytics tools. - Can availability forecasting be applied to all industries?
Yes, while the specific methods may vary, almost all industries—from retail and manufacturing to logistics and healthcare—can benefit from applying availability forecasting to improve their operational efficiency and strategic planning. - What future trends should businesses watch in the field of availability forecasting?
Future trends include the integration of real-time data from IoT devices, the increasing use of big data analytics, enhancements in machine learning models, and a growing focus on sustainability and ethical sourcing in forecasting models.
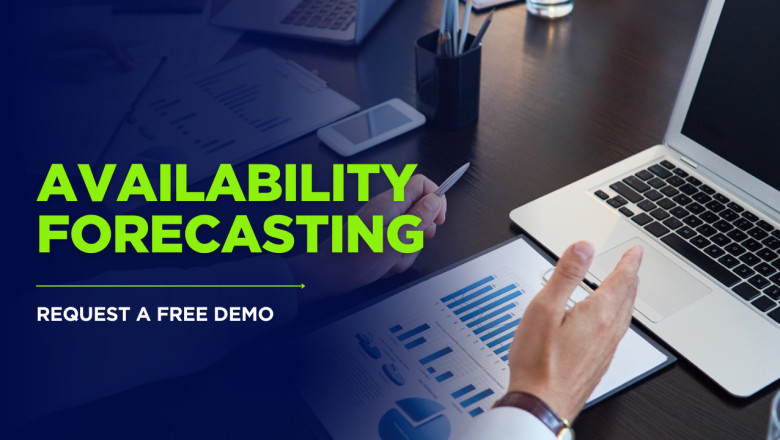

Comments
0 comment