views
Data-Driven Decision Making in the Energy Sector
Energy companies face complex risk environments—from mechanical failures and environmental hazards to volatile market dynamics. Yet many decisions still hinge on intuition or historical precedent rather than forward-looking data analysis. Predictive analytics offers a powerful complement to traditional expertise by using algorithms to detect patterns in large datasets, anticipate emerging issues, and guide proactive measures. This whitepaper explores the role of predictive analytics in elevating risk management, citing practical applications and demonstrable outcomes in the energy industry.
The Rationale for Predictive Analytics
Predictive analytics involves advanced statistical models and machine learning techniques to forecast future probabilities. For example, analyzing years of data on equipment failures can reveal hidden precursors—such as temperature spikes or vibration signatures—that predict a high likelihood of breakdown. In the energy domain, these insights can translate into prescriptive maintenance schedules or optimized asset management, ultimately minimizing costly unplanned downtime.
A review by McKinsey found that predictive maintenance in the energy sector can reduce maintenance costs by up to 20% and equipment downtime by up to 50%. Such benefits extend beyond physical infrastructure to areas like commodity trading, where algorithms forecast price trends based on historical demand, weather patterns, and market signals.
Key Use Cases
- Equipment Failure Prediction: Wind turbine gearboxes or oil pipeline pumps often have sensor arrays that record temperature, pressure, and vibration. Analytics models trained on historical failures learn which sensor patterns typically precede a fault. When a similar signature emerges in real time, the system flags the asset for immediate inspection. This early warning can prevent more extensive damage and safety incidents.
- Reservoir Modeling and Production Optimization: In upstream oil & gas, predictive analytics helps engineers identify where to drill and how to optimize production from existing wells. By correlating seismic data, drilling logs, and production histories, algorithms refine reservoir models, enabling more accurate predictions of reservoir pressure or fluid composition. Some operators report a 5–10% increase in total recoverable reserves after employing advanced analytics.
- Power Demand and Load Forecasting: Utilities rely on weather forecasts, historical consumption patterns, and real-time grid data to predict short-term load. Accurate load predictions reduce the risk of blackouts and help plan energy trades.
- Price and Market Risk: Commodity traders or power producers can model market movements using historical price data, fundamental factors (like gas storage levels), and macroeconomic indicators. Predictive analytics can guide hedging strategies or contract negotiations, stabilizing revenue in volatile markets.
- HSE Risk Analysis: By analyzing near-misses, incident logs, and sensor data (e.g., worker location tracking, environmental conditions), predictive models can highlight tasks or times that carry higher accident risk. A real-world example: a major refinery used machine learning on years of incident records and weather data to find that the majority of slip-and-fall incidents occurred in the early morning when humidity was high. The site introduced targeted interventions (special floor coatings, an early morning toolbox talk) that cut these accidents by 40%.
Technical Prerequisites
Organizations aiming to leverage predictive analytics need robust data pipelines, from sensor networks to integrated databases. Data quality must be ensured—machines can’t learn from incomplete or inaccurate records. Skills in data science, domain knowledge, and a suitable analytics platform are also critical. Many energy companies partner with specialized SaaS providers or build dedicated data science teams. The transition can be gradual: starting with a pilot (e.g., using historical pump data to predict failures) before scaling across all assets.
Risk Management Benefits
Predictive analytics improves risk management by offering:
- Early Warnings: Instead of reacting to failures, companies can schedule proactive interventions.
- Optimized Resource Allocation: Time and budget are directed at assets or areas with the highest likelihood of issues.
- Reduced Insurance and Compliance Costs: Demonstrating predictive maintenance and risk analytics can lower insurance premiums and bolster compliance records.
- Enhanced Safety Outcomes: Forecasting accident risk or equipment malfunctions can avert harmful incidents.
- Continuous Improvement: Models learn over time, refining their predictions as more data is collected.
A North Sea operator reported a 25% reduction in operational incidents following the deployment of a predictive risk management framework that correlated sensor readings with near-miss data. While the initial investment was non-trivial—hiring data scientists and integrating new software—the payoff was substantial in fewer shutdowns, safer conditions, and avoided repair costs.
Get More Information About Services Renewable Energy Industry@ https://toolkitx.com/renewable-energy.html
Conclusion
Predictive analytics ushers in a data-driven future for energy, where decisions are guided by statistical evidence rather than best guesses. Whether it’s scheduling turbine maintenance or predicting price swings, such analytics can substantially reduce risk and volatility. Implementing these capabilities, however, requires more than software: it calls for a cultural shift in how data is collected, shared, and valued within the organization. Early adopters consistently report significant ROI, demonstrating that predictive analytics can be a competitive differentiator in an industry where margin control and operational reliability are paramount.
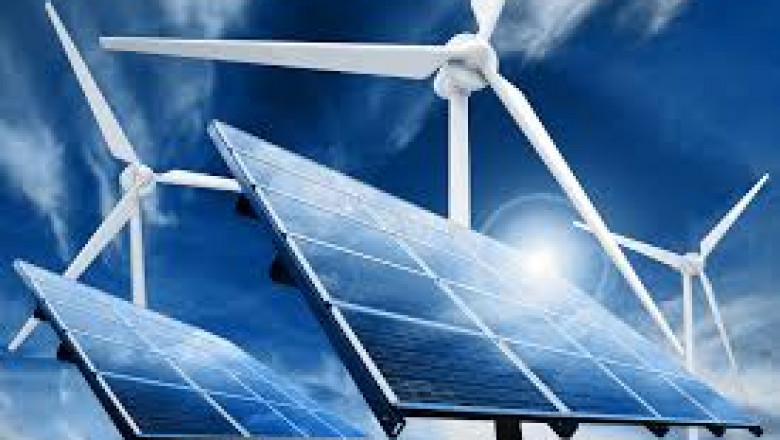

Comments
0 comment